The Role of Machine Learning in Drug Discovery
30 Oct, 20236 minutesThe Role of Machine Learning in Drug Discovery The drug discovery process is a complex ...
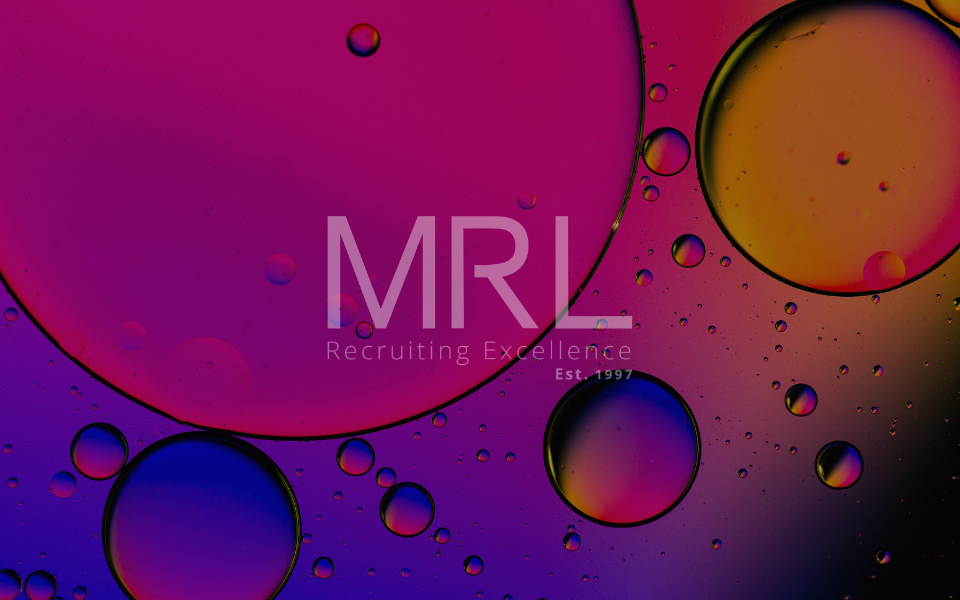
The Role of Machine Learning in Drug Discovery
The drug discovery process is a complex and time-consuming endeavor. It involves the identification of potential compounds, rigorous testing, and meticulous analysis to ensure the safety and efficacy of new medications. However, with the rapid advancement of technology, the landscape of drug discovery is undergoing a remarkable transformation. Machine learning, a powerful tool in the realm of artificial intelligence, is playing a pivotal role in this revolution.
Understanding Machine Learning in Pharma
Machine learning, in the context of pharmaceuticals, is the science of teaching computers to learn and make predictions from data. It's the art of harnessing the immense potential of algorithms to decipher complex patterns, ultimately expediting the drug discovery process.
In today's pharmaceutical industry, where data is abundant, the integration of machine learning is becoming increasingly critical. The synergy between data and machine learning empowers researchers to explore new frontiers in drug development.
Tip: You can learn more about how computer science and automation can benefit the pharmaceutical industry in our articles.
How Machine Learning Can Be Implemented in Drug Discovery
The implementation of machine learning in drug discovery is multifaceted. It encompasses a range of applications that have the potential to revolutionize the industry.
Predictive modeling, for instance, is a fundamental component. Machine learning algorithms can predict the biological activity of compounds, allowing researchers to focus their efforts on the most promising candidates. In addition, these algorithms can identify potential drug candidates by analyzing chemical structures and properties.
Machine learning also plays a crucial role in predicting drug-drug interactions, an area that has significant implications for patient safety. By sifting through extensive datasets, algorithms can flag potential interactions, ensuring that patients receive the most suitable treatments.
Real-world examples abound, showcasing the successes of machine learning in drug discovery. From improved target identification to enhanced lead optimization, the possibilities are expanding rapidly.
Benefits and Advantages of Machine Learning Methods
The advantages of incorporating machine learning in the pharmaceutical industry are profound. It accelerates research and innovation by drastically reducing the time it takes to bring a drug from concept to market. This acceleration not only saves lives but also reduces costs, making healthcare more accessible to all.
Moreover, machine learning and computational drug discovery enhance the efficiency of the drug discovery process. It streamlines data analysis, enabling researchers to focus their attention on the most promising avenues, saving both time and resources.
The applications of machine learning in pharma range in context and methodology, but there can be no doubt - even the deep learning alone that machine learning can extract from biomedical data is worth expanding into.
Challenges and Limitations of incorporating machine learning in drug discovery
As with any transformative technology, the incorporation of machine learning in drug discovery is not without its challenges. Issues such as data quality and interpretability of algorithms present hurdles. Regulatory bodies are also navigating the evolving landscape of computational biology, working to establish guidelines for machine learning applications in pharmaceuticals.
Nevertheless, the pharmaceutical industry is resilient, and ongoing research is dedicated to addressing these challenges. The promise of machine learning in drug discovery is too significant to ignore.
Future prospects of machine learning for drug discovery
The future of machine learning in drug discovery is incredibly promising. It opens the door to personalized medicine, where treatments are tailored to individual patients based on their genetic makeup and specific needs. As machine learning tools become more sophisticated, the potential for targeted, effective treatments will continue to grow.
With the wealth of data generated in healthcare, the field of machine learning is poised for continued growth and evolution. As new algorithms are developed and validated, the possibilities for drug discovery expand exponentially.
Applications of Machine Learning in Drug Discovery nd Development
Here are some key applications of machine learning in this field:
- Drug Target Identification: Machine learning can analyze biological data to identify potential drug targets, such as proteins or genes associated with a disease. This helps researchers focus their efforts on the most promising avenues.
- Drug Repurposing: ML models can predict how existing drugs might be used to treat new diseases by analyzing existing data and identifying potential candidates for repositioning.
- Chemoinformatics: Machine learning can assist in the design of new drug compounds by predicting their properties, activity, and toxicity based on chemical structures and properties.
- Virtual Screening: ML algorithms can be used to virtually screen large chemical libraries to identify potential drug candidates, reducing the number of compounds that need to be synthesized and tested in the lab.
- Pharmacokinetics and Pharmacodynamics (PK/PD): ML models can predict how drugs are absorbed, distributed, metabolized, and excreted in the body, as well as their effects on the target, to optimize dosing and minimize side effects.
- Drug-Drug Interaction Prediction: Machine learning helps in identifying potential interactions between different drugs, which is crucial for avoiding adverse effects when multiple medications are used simultaneously.
- Clinical Trial Optimization: ML can help in patient recruitment and stratification for clinical trials, making trials more efficient and reducing costs.
- Biomarker Discovery: Machine learning can identify potential biomarkers that can be used to diagnose diseases, predict treatment responses, and monitor patient outcomes.
- Adverse Event Detection: ML can be used to monitor real-world data, such as electronic health records, to detect and assess adverse events associated with drugs. The quantitative structure of data makes machine learning the ideal option.
- Genomic Medicine: ML models can analyze genetic data to personalize treatment options, matching drugs to a patient's genetic profile.
- Drug Formulation and Delivery: ML can assist in optimizing drug formulations and delivery methods, making them more effective and patient-friendly.
- Drug Manufacturing: Machine learning can optimize the drug manufacturing process, ensuring quality control and reducing production costs.
- Drug Safety and Pharmacovigilance: ML models can analyze data from various sources to monitor and ensure the safety of drugs on the market, including detecting adverse effects and assessing long-term safety.
- Drug Pricing and Market Access: Machine learning can be used for pricing strategies and assessing the market access and reimbursement landscape for new drugs.
- Drug Combination Therapy: ML can help identify effective combinations of drugs for synergistic effects in treating complex diseases like cancer.
Interested in Pursuing a Career in This Area?
With machine learning, we are not just discovering drugs; we are uncovering a new era in healthcare, filled with hope, innovation, and boundless potential. If you’re interested in entering this industry, look no further. At MRL, we specialize in collaborating with top pharmaceutical companies and key players in the life sciences field. Whether you're seeking your next career challenge or exploring exciting job opportunities in this rapidly evolving industry, our dedicated team of recruiters is committed to supporting you throughout the process. Get in touch with us today and discover how we can assist you in achieving your professional aspirations.